Introduction: The AI Boom and Its Energy Impact
The entire world is witnessing the AI boom, and the demand is skyrocketing, but how it’s transforming industries from chatbots that can experience human-like conversations to AI-powered medical development. But there’s a catch—The appetite for AI is mammoth. But it’s not for foodstuff; it’s for power. The explosive demand of interest in generative artificial intelligence has risen in an arms race to develop the technology. The resultant impact is that many high-density data centres will be required, as well as much more electricity to power them. According to the forecasts of Goldman Sachs Research, data centres’ global power demand will surge 50% by 2027 and by as much as 165% by the end of the decade (compared with 2023).
Concern has been raised about the ROI on current and projected AI investment concerning the recent Chinese advancements, specifically the AI model known as DeepSeek. Still, several questions remain unanswered pertaining to DeepSeek’s training, infrastructure, and capability to scale. Vast amounts of computational resources are required to train large AI models and run complex algorithms. That gives a clear picture regarding how much the Data Centre is working overtime, utilizing more power than ever before. An estimate suggests that up to 10% of the world’s electricity could be consumed by AI Data centres by 2030.
That’s a lot of juice! So, the big question is: Without draining the planet, how do we keep AI’s lights on?
Why AI Models Demand So Much Power
Imagine AI is like a super-smart athlete, and like an athlete who requires regular training to remain competitive, AI also needs constant training to stay sharp. The problem? That training (aka machine learning and deep learning) is intense.
01.
Training vs. Inference
Enormous datasets are required in processing and Training AI models (like ChatGPT, DALL·E, or self-driving algorithms), often using thousands of GPUs and TPUs for weeks. After training, the inference still consumes substantial power to use the model to generate responses or predictions.
02.
High-Performance Hardware
Running AI on an average laptop is not the icing on the cake. It requires power-hungry GPUs, TPUs, and AI accelerators, as well as specialized cooling and massive energy inputs.
03.
Data Storage & Processing
AI models prosper on data—petabytes of it! Another layer of energy utilization is added to store and transfer this data across the cloud.
04.
24/7 Operations
Many AI-driven applications that run non-stop, like fraud detection, voice assistants, and recommendation engines, further increase power demands.
The energy consumption problem will only intensify unless we act fast since AI models are growing bigger and bigger at regular intervals (GPT-3 had 175 billion parameters, and its successors are even huge).
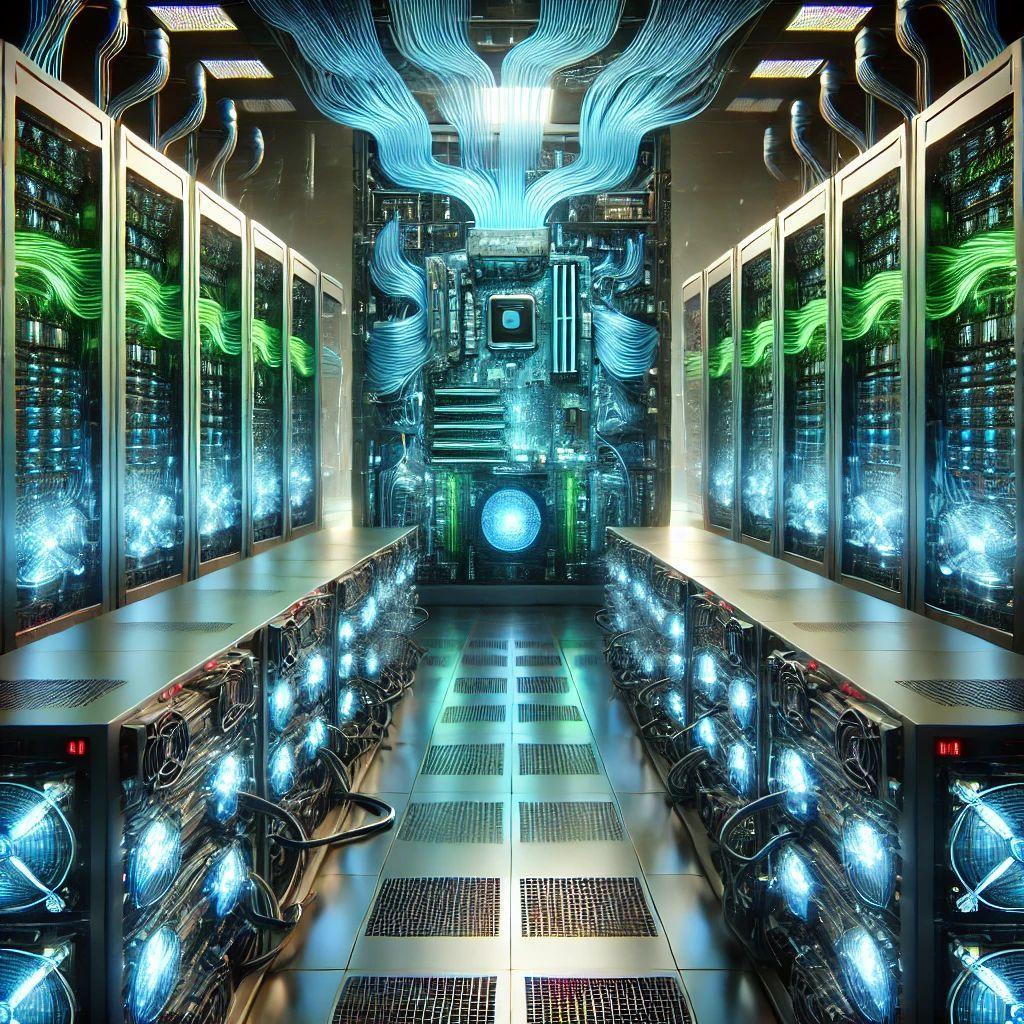
A Futuristic Data Centre
Are There Sustainable Solutions?
The promising news? Companies and researchers are proactively working on greener ways to power AI. Here are some encouraging solutions:
05.
Energy-Efficient AI Hardware
ARM-Based Chips & Neuromorphic Computing: Unlike traditional CPUs and GPUs, which require more energy, these ARM-Based Chips & Neuromorphic Computing architectures mimic the human brain’s efficiency, reducing power consumption.
Specialized AI Chips: Google’s TPUs and NVIDIA’s latest AI accelerators are designed to perform deep learning tasks with significantly lower energy use.
06.
AI-Optimized Data Centers
Liquid Cooling: Companies are incorporating liquid immersion cooling instead of energy-intensive air cooling to keep AI servers from overheating, reducing power waste.
Dynamic Workload Scheduling: Based on real-time demand and to optimize energy usage, AI itself is being used to ensure data centres run efficiently.
07.
Renewable Energy & Carbon Offsets
Solar & Wind-Powered Data Centers: In another move to reduce power consumption, major cloud providers like Google and AWS are swinging toward 100% renewable energy-powered data centres.
Carbon Capture Initiatives: To counterbalance their environmental effect, some companies are investing in carbon offset programs.
08.
Edge AI Computing
Edge computing processes AI tasks closer to users (on local devices or smaller edge servers) instead of transferring all AI workloads to huge cloud data centres, reducing overall power consumption.
Case Studies: Companies Tackling AI’s Energy Consumption
The battle for sustainable AI is on! Here’s how some of the big players in the tech world are tackling the issue:
09.
Google’s DeepMind AI for Energy Optimization
To optimize cooling systems in its data centres, Google has been using AI for energy optimization, reducing energy use by up to 40%. Their target? Aiming to make their cloud platform completely carbon-free by 2030.
10.
Microsoft’s Underwater Data Centers
Microsoft's "Project Natick" explored the feasibility of underwater data centres, finding that the cool ocean temperature naturally reduces the need for energy-intensive cooling systems, leading to lower failure rates and improved energy efficiency.
11.
Meta’s AI-Powered Cooling Systems
To optimize airflow and cooling in its data centres, Meta (formerly Facebook) employs machine learning models, thus minimizing energy waste.
12.
Tesla’s Dojo Supercomputer
An energy-efficient AI training supercomputer, Dojo, has been developed by Tesla and is designed to be more power-efficient than conventional AI training clusters.
Future Trends: The Road to Greener AI
The future of AI is not only about being smarter; rather, it’s more about sustainability. Here’s what’s coming:
13.
AI for AI: Self-Optimizing Systems
Without sacrificing performance, AI models will be trained to augment their energy usage to improve productivity.
14.
The Rise of Quantum Computing
Compared to traditional supercomputers, quantum computers could revolutionize AI by solving complicated problems with less energy.
15.
Hybrid Cloud & Multi-Cloud Strategies
Based on demand, companies will distribute AI workloads across multiple cloud providers by selecting the most energy-efficient option.
16.
AI Regulation & Sustainability Standards
Stricter regulations and carbon accounting standards are expected to push AI companies toward greener practices.
Conclusion: The Balancing Act Between AI Growth and Sustainability
AI is here to be with us, and its energy consumption is bound to grow like anything—but so will our ability to make it sustainable. The tech industry can power AI’s future without draining the planet by spending on energy-efficient hardware, smarter data centre management, and renewable energy sources.
So, do we have a solution?
We’re getting there! With unrelenting innovation, AI’s energy footprint can be minimized while its intelligence soars.
Small changes today can lead to big impacts on the planet tomorrow. What are your thoughts on AI’s energy consumption? Let’s discuss this in the comments!